The Future of Data Science: Navigating the Next Decade of Innovation
How emerging technologies and methodologies will reshape the data landscape
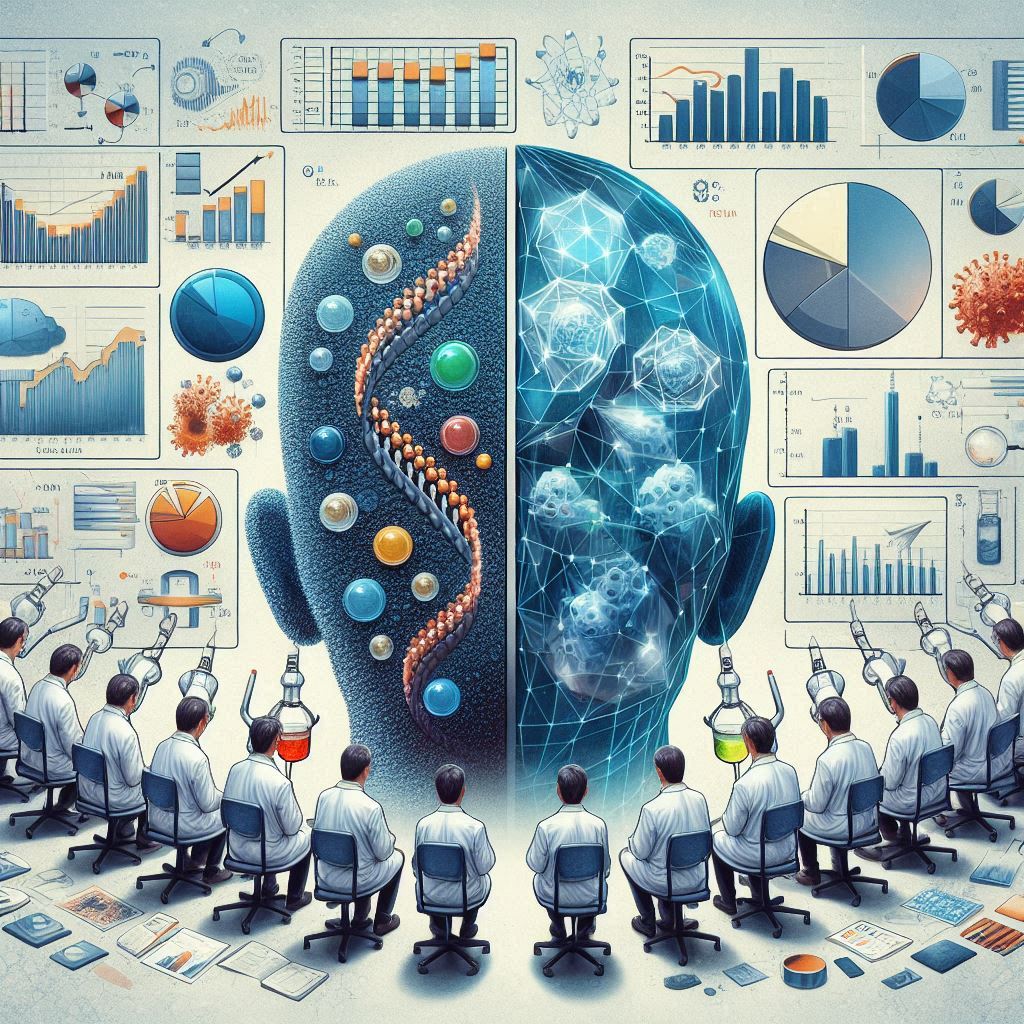
Introduction: The Evolving Data Landscape
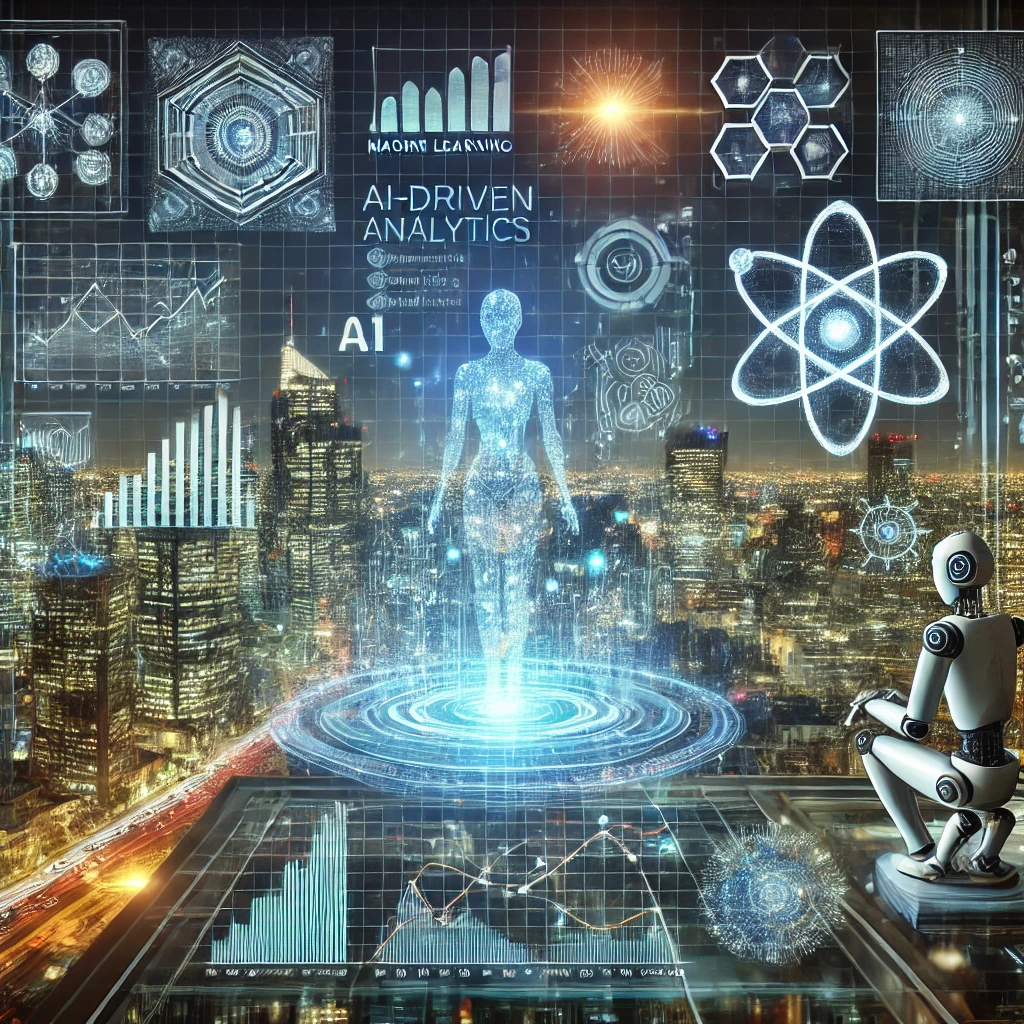
The field of data science stands at a pivotal crossroads. Over the past decade, we've witnessed exponential growth in data generation, storage capabilities, and computational power. Organizations worldwide have embraced data-driven decision-making, recognizing that insights extracted from massive datasets can provide significant competitive advantages. However, as we look toward the next decade, the data science discipline is poised for transformative evolution that will fundamentally reshape how we interact with information and derive value from it.
Currently, global data creation is estimated to exceed 2.5 quintillion bytes daily, a figure expected to grow exponentially with the continued expansion of IoT devices, social media, and digital transactions. This data deluge presents both unprecedented opportunities and formidable challenges for organizations and practitioners. The future of data science will be defined by how effectively we can harness this expanding data universe while addressing emerging ethical, technical, and organizational barriers.
In this comprehensive exploration, we'll examine the key trends, technologies, and methodologies that will define the next decade of data science innovation. From the democratization of analytics to the rise of quantum computing, these developments promise to expand the boundaries of what's possible in data-driven discovery and decision-making.
AutoML and the Democratization of Data Science
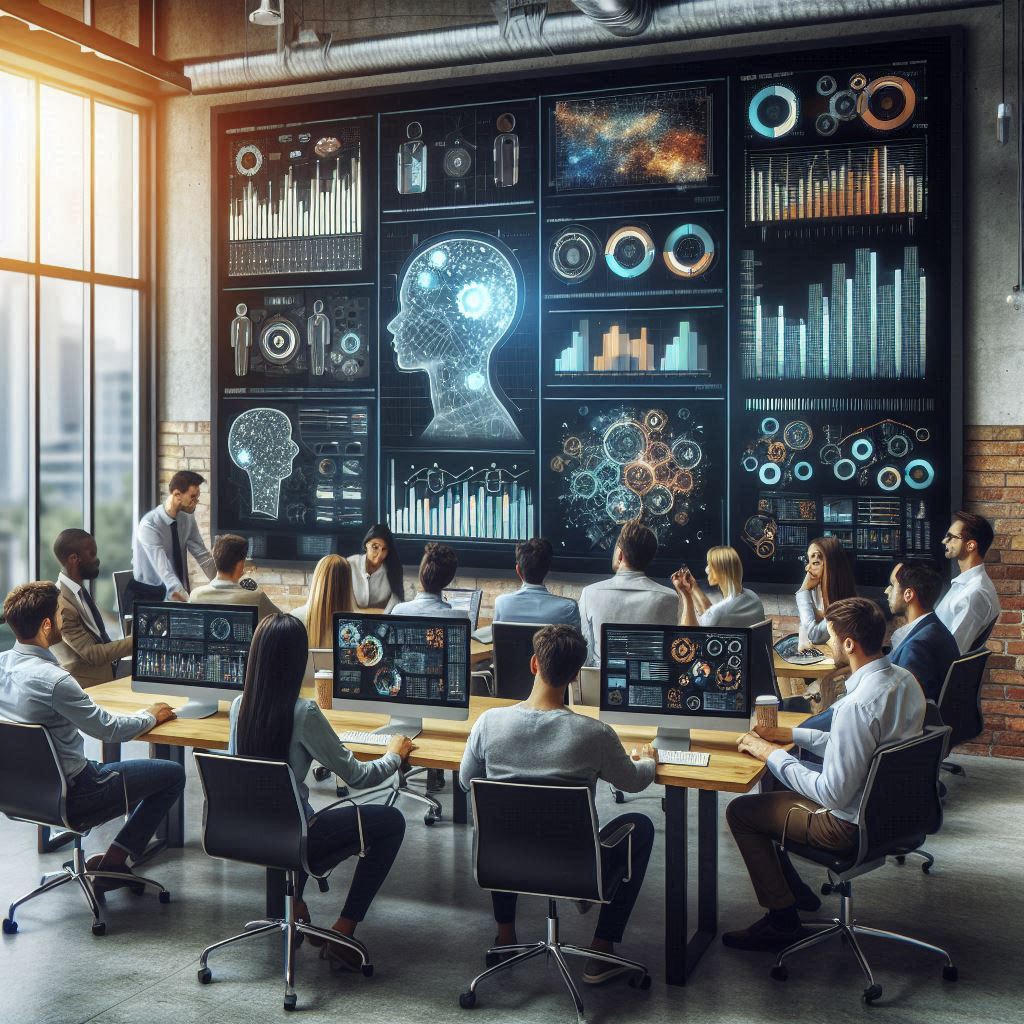
One of the most significant trends reshaping the data science landscape is the rise of Automated Machine Learning (AutoML). These systems are designed to automate the end-to-end process of applying machine learning to real-world problems, from data preprocessing and feature engineering to model selection and hyperparameter tuning.
The implications of AutoML extend far beyond mere efficiency gains for existing data scientists. By removing technical barriers to entry, these tools are democratizing access to sophisticated analytics capabilities. Domain experts in fields like healthcare, finance, and marketing can now leverage machine learning without requiring deep technical expertise in statistical modeling or programming. This democratization effect is poised to expand dramatically in the coming decade, as AutoML platforms become more capable, intuitive, and integrated into business workflows.
The evolution of AutoML will likely follow several parallel paths. First, we'll see continued advances in the automation of increasingly complex tasks, including feature creation and deep learning architecture design. Second, these platforms will become more accessible through natural language interfaces and visual programming environments. Finally, specialized AutoML systems will emerge for particular domains and use cases, incorporating domain-specific knowledge and best practices.
However, this democratization raises important questions about the changing role of data scientists. Rather than being replaced, human experts will likely shift toward more strategic functions – defining problems, interpreting results, ensuring ethical implementation, and handling the most novel or complex analytical challenges that remain beyond automation's reach.
Edge Analytics and Distributed Intelligence
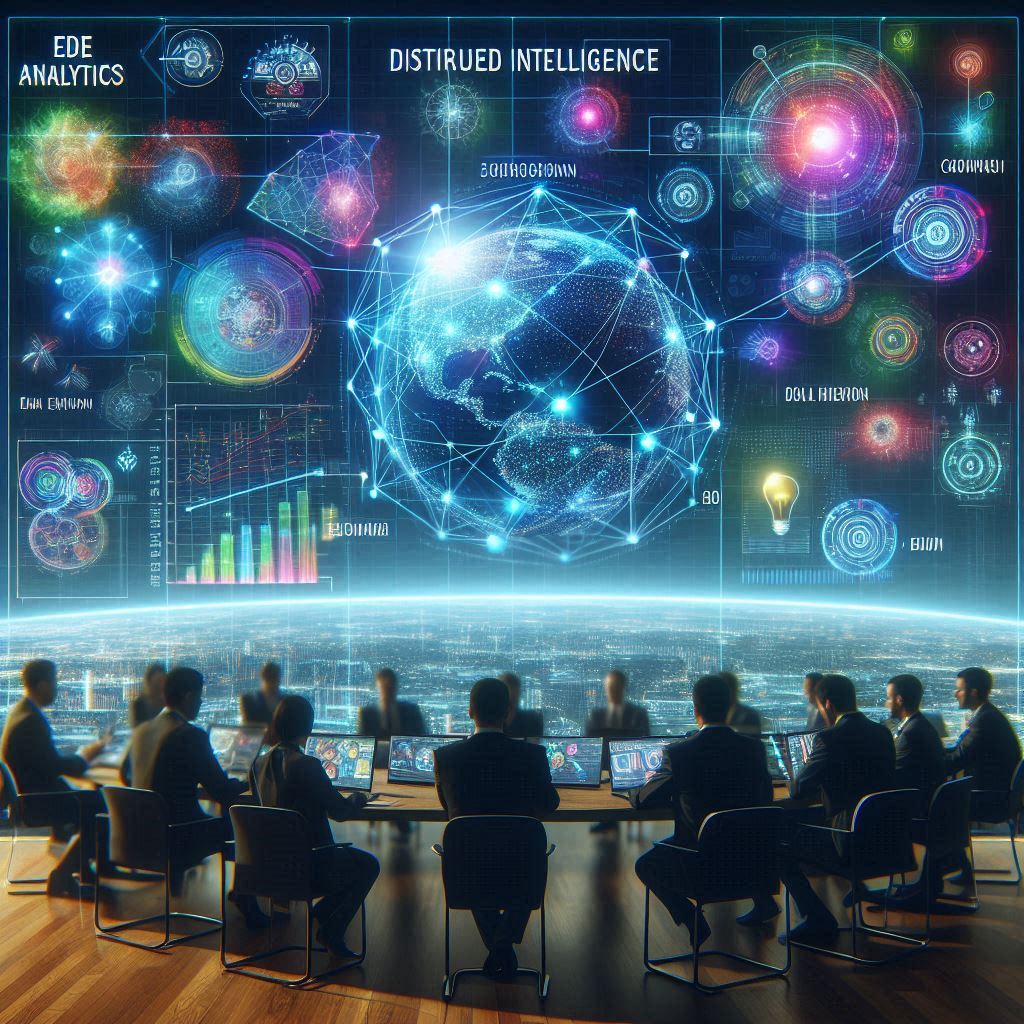
The traditional paradigm of centralizing data in cloud repositories or data warehouses for analysis is facing significant challenges. As IoT devices proliferate and real-time decision requirements increase, the latency and bandwidth limitations of cloud-centric approaches become increasingly problematic.
Edge analytics – processing data closer to where it's generated rather than transmitting it to centralized systems – represents a fundamental shift in data architecture that will gain prominence in the coming decade. By 2025, Gartner predicts that 75% of enterprise-generated data will be created and processed outside traditional centralized data centers.
This shift toward distributed intelligence will necessitate new approaches to model deployment, monitoring, and governance. Federated learning techniques, which allow models to be trained across multiple edge devices without exchanging the underlying data, will become increasingly important for privacy-sensitive applications. Similarly, techniques for model compression and optimization will evolve to enable sophisticated analytics on resource-constrained edge devices.
The implications for data science practice are profound. Rather than working with static, centralized datasets, practitioners will increasingly design distributed analytics ecosystems where intelligence is embedded throughout the data supply chain. This shift will require new skills and toolsets focused on distributed systems, real-time analytics, and embedded AI.
Causal AI and Explainable Machine Learning
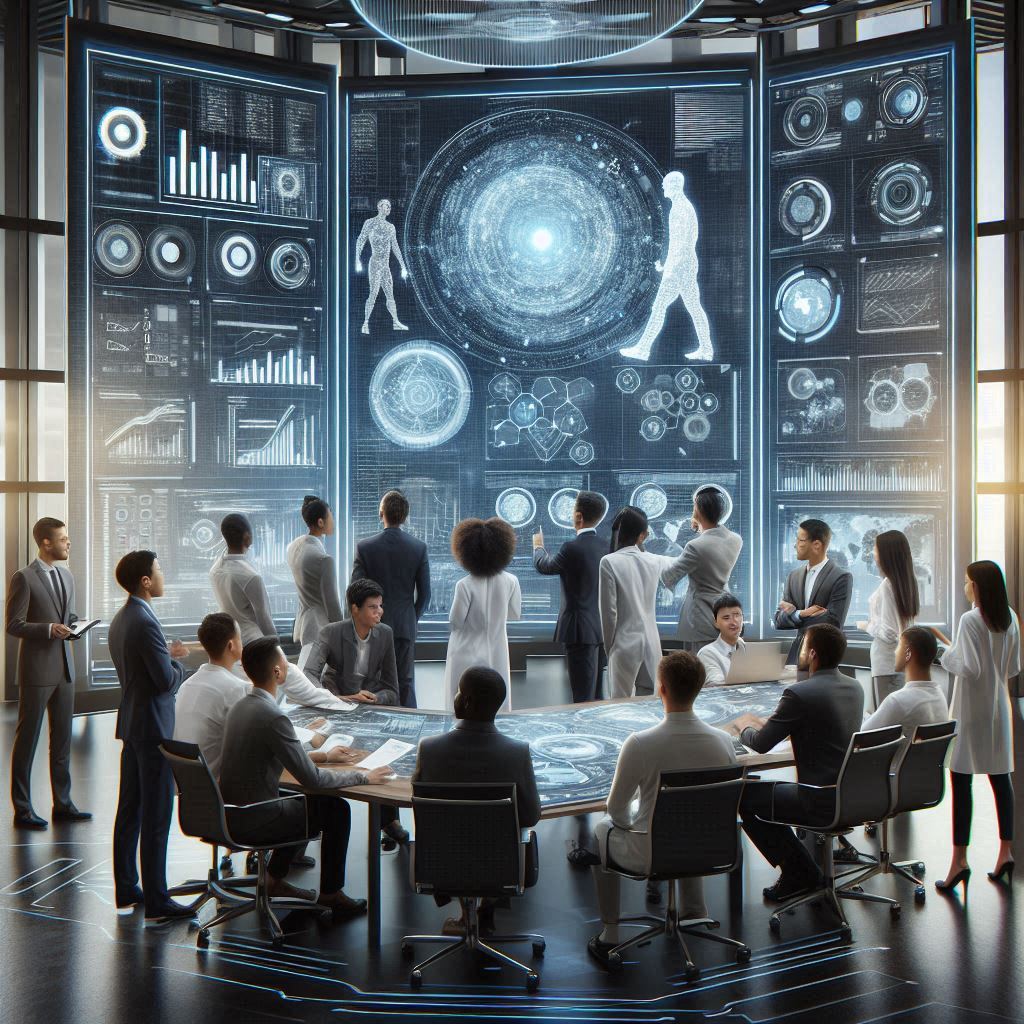
As machine learning systems increasingly inform critical decisions in areas like healthcare, finance, and criminal justice, the limitations of purely correlational approaches have become apparent. The next frontier in data science involves moving beyond pattern recognition toward causal understanding – identifying not just what happens, but why it happens and what interventions might change outcomes.
Causal inference methods, which have historically evolved separately from mainstream machine learning, are now being integrated into modern AI systems. These techniques allow models to reason about counterfactuals, estimate treatment effects, and better handle distribution shifts. Judea Pearl's causal hierarchy – progressing from association to intervention to counterfactuals – provides a useful framework for understanding this evolution.
Concurrently, the field of explainable AI (XAI) continues to advance, developing methods to make complex models more interpretable without sacrificing performance. Techniques like SHAP (SHapley Additive exPlanations) values, integrated gradients, and attention visualization are becoming standard components of the machine learning toolkit.
In the coming decade, these parallel trends of causal AI and explainable machine learning will likely converge, yielding systems that can both identify causal relationships and explain their reasoning in human-understandable terms. This convergence will be particularly important in regulated industries where algorithmic decisions must be justified to stakeholders and auditors.
Quantum Computing and Next-Generation Analytics
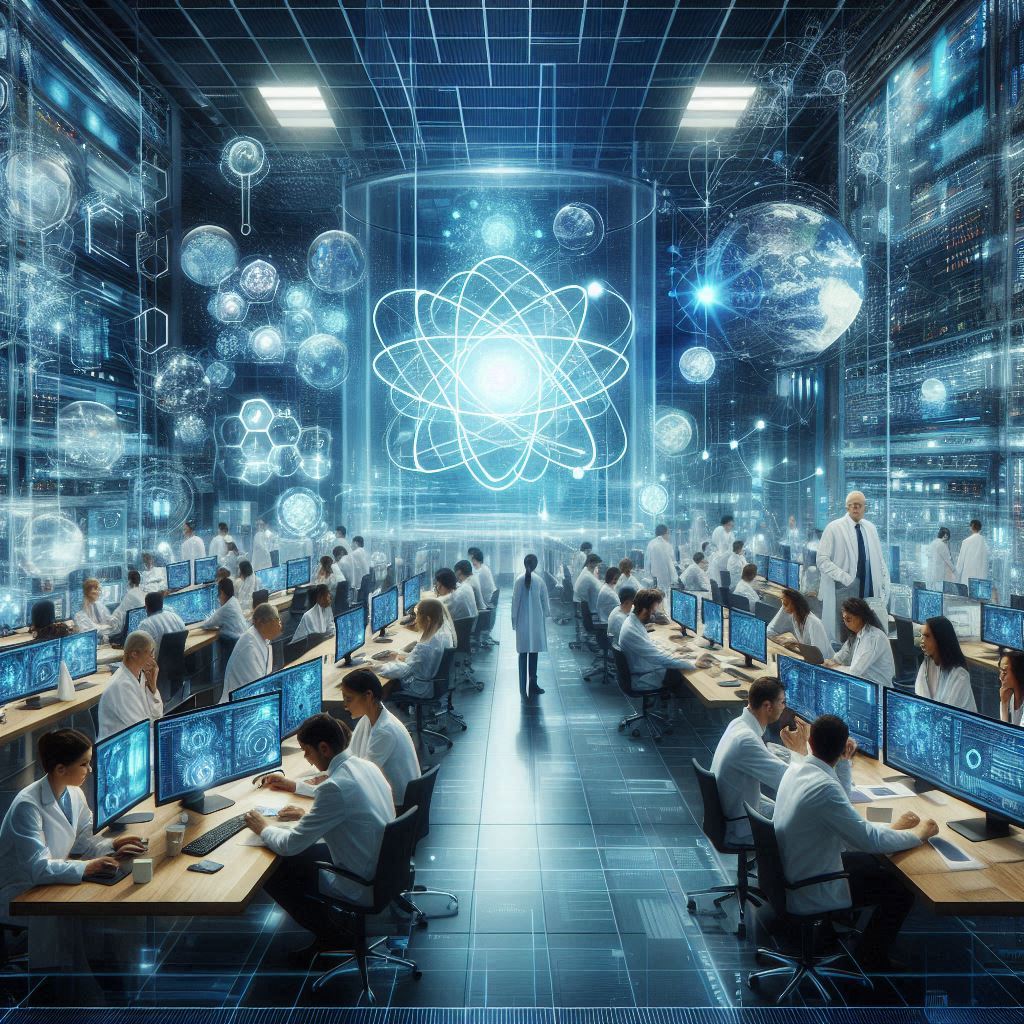
Perhaps no emerging technology holds more transformative potential for data science than quantum computing. While still in its early stages, quantum computation promises to revolutionize how we approach certain classes of problems that remain intractable even for today's most powerful supercomputers.
Quantum machine learning (QML) represents the intersection of quantum computing and data science. Certain quantum algorithms can theoretically provide exponential speedups for specific machine learning tasks, particularly those involving high-dimensional data or complex optimization problems. For instance, quantum principal component analysis and quantum support vector machines might enable analysis of datasets far beyond the capabilities of classical systems.
The timeline for practical quantum advantage in data science applications remains uncertain, with estimates ranging from 5 to 15 years. However, the field is advancing rapidly, with major technology companies and startups making significant investments in quantum research and development.
Forward-thinking data science organizations should begin preparing for the quantum era by identifying potential use cases that align with quantum's strengths, experimenting with quantum-inspired classical algorithms, and developing expertise in quantum programming frameworks like Qiskit and PennyLane. The transition to quantum-enhanced data science will likely be gradual, with hybrid classical-quantum approaches serving as an important bridge technology.
Ethical AI and Responsible Data Science
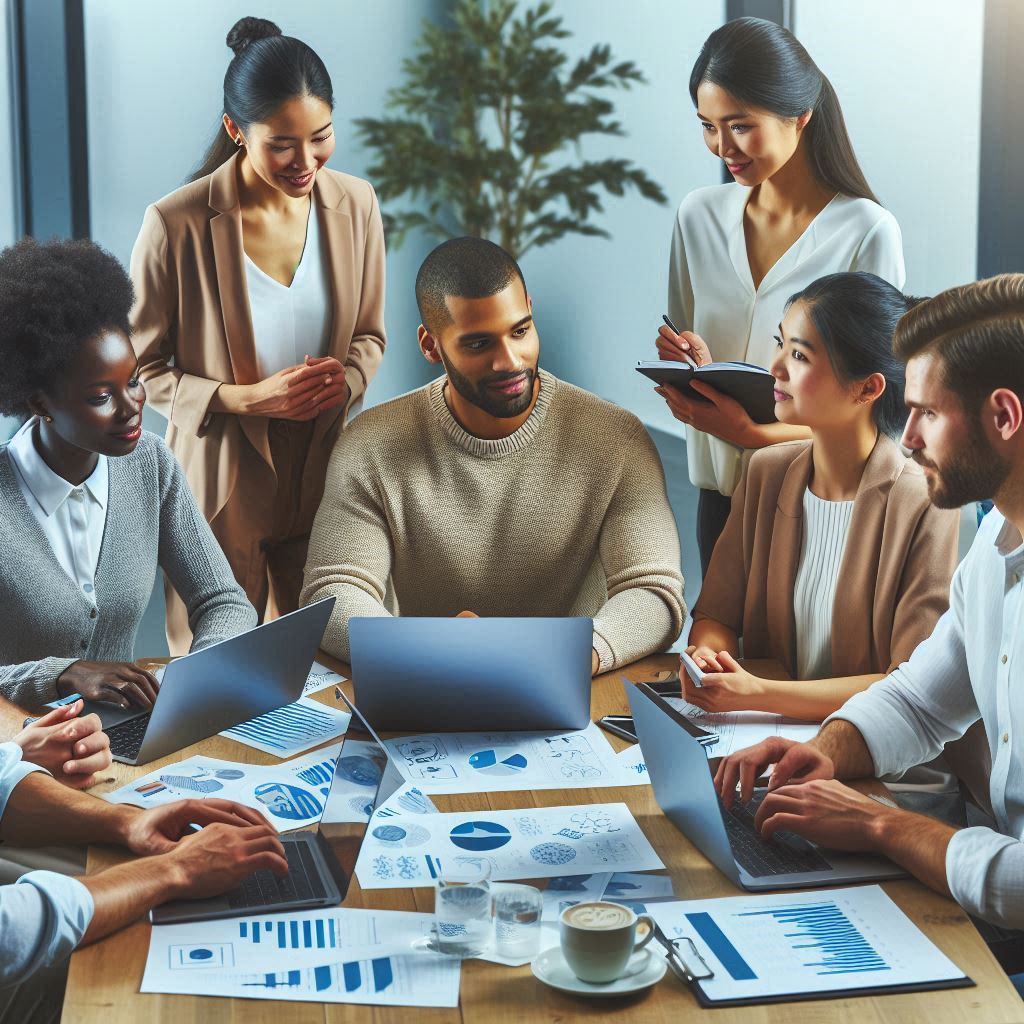
As data science capabilities become more powerful and pervasive, questions of ethics, fairness, and responsibility have moved from academic discussions to urgent practical concerns. High-profile controversies involving algorithmic bias, privacy violations, and unintended consequences of AI systems have highlighted the risks of deploying sophisticated analytics without adequate safeguards.
The next decade will see the continued maturation of responsible data science as both a technical discipline and organizational practice. From a technical perspective, methods for detecting and mitigating bias, ensuring differential privacy, and implementing algorithmic fairness will become more sophisticated and integrated into standard workflows. From an organizational perspective, frameworks for AI governance, model risk management, and ethical review will become standard components of the data science lifecycle.
Regulatory attention to AI and automated decision systems is also increasing globally, with landmark legislation like the EU's General Data Protection Regulation (GDPR) and the proposed AI Act establishing new requirements for transparency, explainability, and human oversight. Data scientists will increasingly need to demonstrate compliance with these evolving regulatory frameworks.
Perhaps most fundamentally, the conception of data science success will expand beyond technical metrics like accuracy and efficiency to include broader considerations of social impact, inclusivity, and alignment with human values. This evolution represents not a constraint on innovation but rather a recognition that truly successful data science must serve human flourishing in its fullest sense.
Conclusion: Preparing for the Future
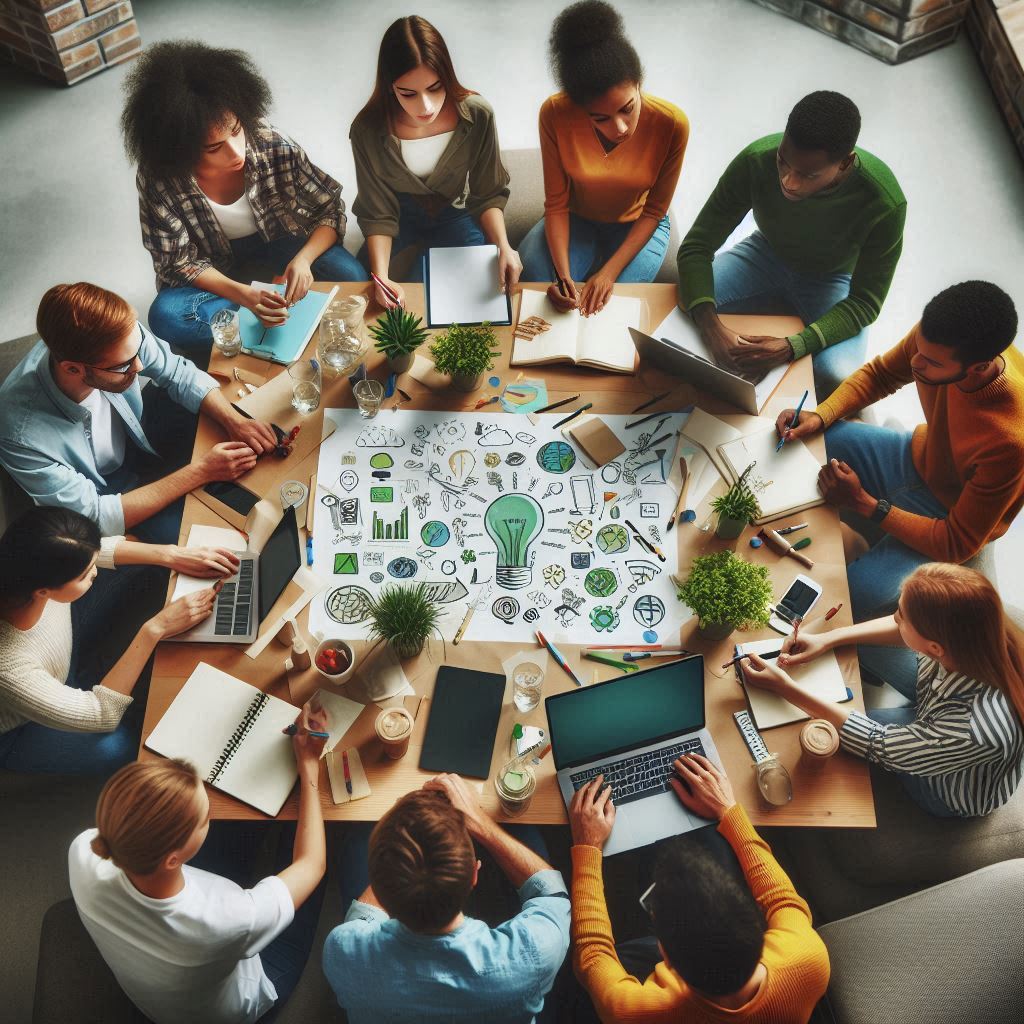
The future of data science will be shaped by the convergence of technological innovation, methodological advances, and evolving societal needs. Organizations and practitioners that can anticipate these trends and adapt accordingly will be best positioned to harness the transformative potential of data in the coming decade.
Successful navigation of this future requires both technical agility and strategic foresight. Technical teams must continuously expand their capabilities, embracing new tools and methodologies as they emerge. At the same time, organizational leaders must develop clear data strategies that align analytics capabilities with business objectives and ethical principles.
Perhaps most importantly, the future of data science depends on cultivating diverse talent and perspectives. The most challenging problems in the field – from algorithmic fairness to causal inference – require not just technical expertise but also deep understanding of social contexts and human factors. By bringing together individuals with varied backgrounds, experiences, and ways of thinking, the data science community can develop more robust, innovative, and responsible approaches to data-driven discovery.
As we stand on the threshold of this exciting new era, one thing is clear: the future of data science extends far beyond technology alone. It is ultimately about expanding human capability and insight through the thoughtful application of data and computation – a mission that will continue to inspire and challenge us in the decades to come.